A safer, more resilient world: Reducing disaster risks with AI
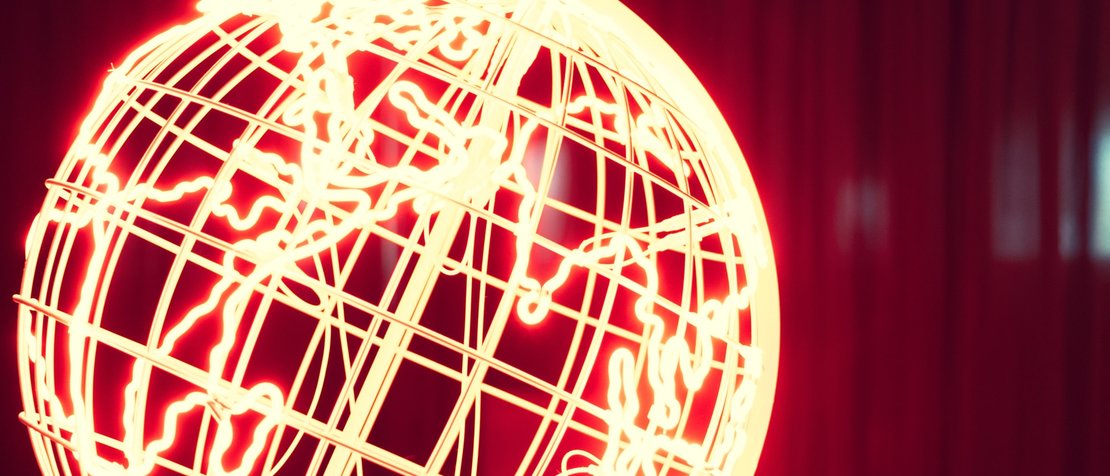
Natural and human-made disasters upend lives and livelihoods around the world on a recurring basis. In a disaster, smart, timely decisions are needed to avert, mitigate and manage all kinds of risks. In this respect, the use of artificial intelligence (AI) in the decision-making process has shown both tremendous promise as well as scope for improvement.
The opportunities and challenges of AI in disaster risk reduction (DRR) were on the agenda of a webinar held in partnership with the UN Environment Programme during the AI for Good Global Summit.
By saving time and rapidly analyzing large amounts of data, artificial intelligence can help make inroads into achieving the UN Sustainable Development Goals and implementing the Sendai Framework for Disaster Risk Reduction 2015-2030.
How AI enhances geospatial mapping
Artificial intelligence leverages remotely sensed geospatial data from satellites to detect and mitigate potential disasters, and its use in damage mapping and image recognition saves lives during emergencies.
Combining AI with hardware has also led to disaster relief robots and drones that deliver aid in risky situations, noted Bilel Jamoussi, Chief of the Study Groups Department at ITU’s Telecommunication Standardization Bureau.
“ITU’s publication ‘Disruptive technologies and their use in disaster risk reduction and management‘ puts the spotlight on AI as a new and emerging technology that can hasten the spread of critical information, improve understanding of disasters, enhance early warning systems, assess damage in new ways and add to the knowledge base of social behaviours and economic impact after a crisis,” Jamoussi said.
Understanding and quantifying disaster risk requires accessible and detailed up-to-date geospatial data that traditional urban collection methods do not provide, said Yves Barthélemy, Senior Geospatial and Resilience Specialist at the World Bank. For example, to assess earthquake risks accurately, there is a need to know where and how people live along with information about buildings.
Barthélemy’s team embarked on the world’s largest drone mapping exercise in Zanzibar using local drone pilots. “We used the drone imagery and extracted the building roof print automatically using drone images and machine learning,” he said.
The results gave details about the condition of buildings and their state of construction, which were critical in understanding earthquake and flood risks.
Scalable AI for faster impact
Sella Nevo, a Senior Software Engineer who leads the Google Flood Forecasting Initiative, believes that technology must be computationally scalable and sustainable to have an impact. His team has been working on AI for actionable warnings and forecasts for riverine floods.
To forecast water levels throughout a river, Google has used models based on LSTM (Long Short-Term Memory) a neural network architecture specialized for time series that provide more scalable results. “They can be trained across hundreds of sites with a single click as opposed to classical models which need to be calibrated manually,” said Nevo.
Available data sometimes do not account for real-time events on the ground.
To fix this, Nevo and his team combined a machine learning model estimating the profile of the river with a physics-based model to expand the profile to a two-dimensional floodplain.
“Reducing data latency, detecting and correcting errors in data collection and increasing the speed and frequency at which these models are run is perhaps less sexy but critically important to take these models out of academia and allowing them to create change on the ground,” Nevo said.
Reducing risks with open and coordinated data
Researchers at the Monash Data Futures Institute in Australia have used data sourced from the European Space Agency’s Sentinel-2 satellite and analyzed more than 4,300 images to develop a high-resolution vegetation map of the state of Victoria.
The moisture in soil and atmospheric temperatures are key drivers of bushfire risks. Using a time series classification, scientists built models and annotated data to better understand vegetation cover and how land is being used for different purposes.
“These maps have the potential to assist with bushfire prevention, agricultural planning, flood modelling, pollution management and rehabilitation,” said Joanna Batstone, the institute’s Director.
Batstone emphasized the need for standardization of approaches and data sets, and better methods to access data that is already available.
“Many disaster risk reduction approaches today rely on single data streams such as assessing rainfall, temperatures or vegetation. We need to bring those data sets together,” she highlighted.
“Not just input data, but data output from models should also be open,” according to the World Bank’s Barthélemy. Opening code libraries and training data sets will democratize the disaster risk management process so that more people can use it, he added.
AI in DRR: A double-edged sword
Despite its promise, Jamoussi acknowledged that AI in disaster management is a “double-edged sword” with problems of data access, data bias and data manipulation.
Lack of access to telecommunications networks is also a barrier in implementing AI solutions, he added. ITU is working with the World Food Programme’s Emergency Telecommunication Cluster to develop a disaster connectivity map which will use disruptive technologies to identify better ways of understanding connectivity gaps following disasters.
Adam Fysh, Programme Management Officer at the United Nations Office for Disaster Risk Reduction (UNDRR), suggested that AI could potentially demonstrate the benefits of opening data that is currently locked away in ministries, institutions and communities.
Co-designed approaches needed
Fysh also invited the AI community to participate in a co-designed approach, taking the concerns and priorities of those working on DRR into account.
For instance, rather than flood forecasts, their priority might be cheaper infrastructure repairs or improving education outcomes that suffer due to schools being deployed as emergency shelters. He noted that AI has the capacity to stress-test different scenarios and understand what the influencing factors are for risk rather than trying to anticipate specific events.”
“AI has the capacity to stress-test different scenarios and understand what the influencing factors are for risk rather than trying to anticipate specific events.”
Fysh called for more people to participate in the DRR process as risks can be constructed by anyone. He also felt it would help if more people understand and question risk information. “This is a capacity building job for the AI community that’s not related to the accuracy of computations but about building the cohort of people that you’re trying to help,” he said.
Towards local community engagement and inclusive methodologies
Along with advances in the use of technology, engaging with local people and local contexts to inform data and share results is also important for DRR.
In populated regions with fewer resources, solutions have to be simple, cost-effective, easily deployed and developed locally for DRR strategies to have long-lasting impact.
“AI applications together with citizen science methods to empower community engagement can help build a local understanding of the potential impacts of disaster and empower communities to take action in reducing risks,” said Batstone.
Preeti Banzal from the Office of the Principal Scientific Adviser to the Government of India sees potential for AI in post-disaster needs assessment. During the floods in Chennai and Kochi in southern India, big data analytics and AI were deployed to extract information from social media and used for response and relief.
She called for AI to be responsible, sustainable and inclusive.
“Using Natural Language Processing (NLP) for language translation, AI systems can create early warnings simultaneously in many vernacular languages in a country like India which has about 20,000 languages and dialects,” Banzal said.
AI-enabled apps can warn and guide people with disabilities during impending disasters, she added.
The vital role of standardization
There is a need to create standardization for the use of responsible AI, said Banzal.
In response, Jamoussi raised examples of ITU focus groups that are looking at benchmarking the use of AI for health and autonomous/assisted driving. “In the case of DRR, we could envision having a focus group that is an open platform for members and non-members of the ITU where the scientific community, practitioners on the field, companies and the data holders can come together,” he said.
This group could together look at how AI is being used for DDR and address questions about datasets, APIs and benchmarking of algorithms to produce technical reports and specifications for the world.
ITU standards already offer common formats for the exchange of all-hazard information over public networks, ensuring that emergency communications are prioritized. ITU standards also offer guidance on network architectures able to contend with sudden losses of substantial volumes of network resources. They describe the network functionality required to make optimal use of the network resources still operational after a disaster.
In the case of damaged ICT infrastructure, standards offer techniques for rapid repair, such as means to connect surviving fibres of severed fibre-optic cables. And they provide for “movable and deployable ICT resource units” – emergency containers, vehicles or hand-held kits housing network resources and a power source – to provide temporary replacements for destroyed ICT infrastructure.
Learn more about ITU Emergency Communications Actions in radiocommunications, standardization and development here.
Image credit: gerti gjuzi via Unsplash.