How AI is helping uncover modern slavery
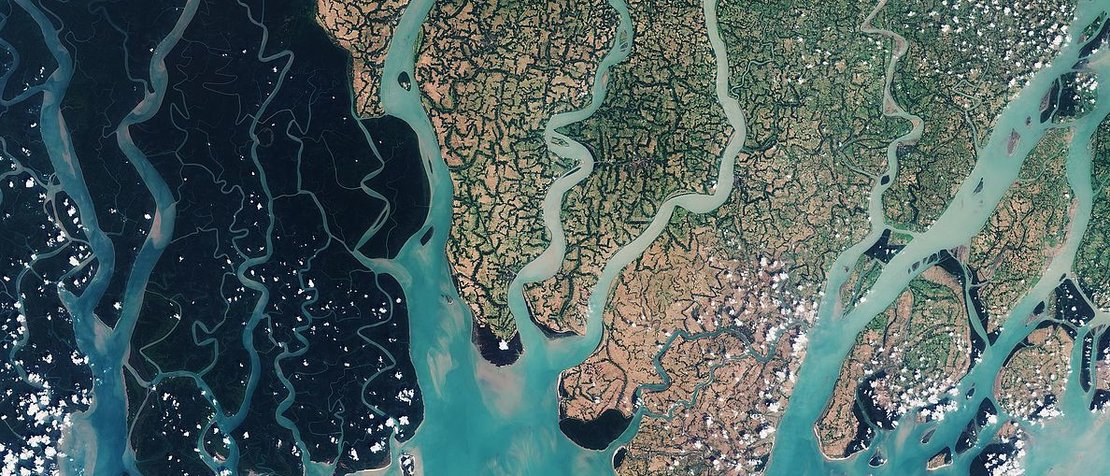
More than 40 million people around the world are enslaved today. Eliminating modern slavery has been particularly challenging due to its near-invisible nature – until recently.
Experts from the Code 8.7 community have turned to artificial intelligence (AI) and computational science to fight modern slavery, along with human trafficking and forced child labour. The group takes its name from Target 8.7 of the United Nations Sustainable Development Goals, asking UN Member States to take “immediate and effective measures” to eradicate modern slavery and end child labour by 2025.
Spotting anomalies and proxy data
The hundreds of millions of dollars that traffickers are estimated to rake in yearly through the modern slave trade may largely be laundered through legitimate financial institutions. “These monies are moved through intricate networks, using financial systems both within and across countries,” noted Anjali Mazumder, an expert in AI, justice, and human rights at The Alan Turing Institute.
Mazumder spoke at a webinar on AI to Prevent Modern Slavery, Human Trafficking and Forced and Child Labour as part of the AI for Good Global Summit, hosted online year-round by ITU and other partners.
To root out traffickers, her team uses network analysis and anomaly detection to identify patterns in financial flows. Law enforcement officials, she notes, often struggle to communicate with financial experts and institutions. Yet AI – combined with privacy-enhancing technologies, such as multi-party computation – eases the flow of data.
Linking to ground truths
James Goulding, Deputy Director of N/LAB at the University of Nottingham and his team tease out ‘ground truths’, or direct observations about vulnerabilities, and combine them with data on mobile money transactions, cell tower activity or transport usage.
“Those datasets help us understand whether we can find proxies that reflect the vulnerability we see in our ‘ground truths’,” he said.
While AI and machine learning help his team find complex non-linear relationships, AI is not just about predicting. It also helps to unpack new explanations and use those insights for further innovation.
Datasets about cell towers and the number of messages exchanged, for example, can indicate activity in a given area. For a project investigating urban slavery in Dar es Salaam Tanzania, such data also “gives an insight to overcrowding and certain types of demographics,” Goulding noted.
“You can see patterns of the communities that exist in this data and use AI to model those patterns.”
A field survey conducted with the World Bank enabled Goulding’s team to connect slavery risk estimates to other datasets for Tanzania. Drone and satellite imagery are also valuable data sources.
“Financial independence can act very much as a protection against enslavement,” he adds. “Areas with increased use of mobile money are less vulnerable to domestic slavery and forced labour.”
Yet other areas where pay was relatively high showed signs of slavery. “People might be drawn to high pay expectations and fall into a trap of forced labour,” Goulding suggested. “The AI model presented examples of an industrial area with job opportunities. People come to the slums nearby, where they are the most vulnerable. That is where resources should be applied.”
Unearthing links with Earth observation data
Goulding’s colleague Doreen Boyd, Professor of Earth Observation (EO) at the University of Nottingham, has studied the use of earth observation satellites as continuous data source.
In Bangladesh’s Sundarbans mangrove forest, “an archive of data to map fish processing camps helped us track their evolution through time,” she said.
Following reports of forced labour at one camp, spatial and temporal analysis based on EO data revealed operational trends. Boyd’s team noted a cyclical relationship between labour practices and ecological degradation, “where environmental damage is both a driver and a result of workers being subjected to modern slavery.”
A study on child labour for cobalt mining in the Democratic Republic of Congo relied on data fusion, with Interferometric Synthetic Aperture Radar (InSAR), a form of high-resolution EO data, indicating ground movements that indicated tunnelling. Machine learning then helped to map the tunnels.
Such data needs still needs to be better understood and labelled. “It’s still very much in its infancy,” Boyd said.
“We still require ground intelligence to tell us what we need to extract from the EO data.”
Nadya T. Bliss, Executive Director of the Global Security Initiative at Arizona State University, stressed the need to combine AI with expertise from different fields. “This notion of lived experience is also vital to bring in.”
Join Code 8.7’s effort to achieve Target 8.7 by becoming a member here.