How machine learning is powering collective pandemic intelligence
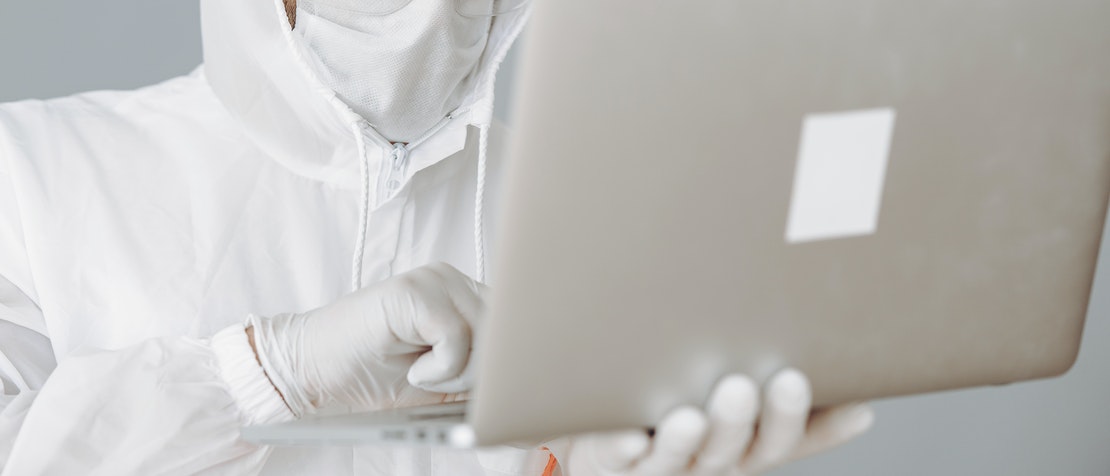
As the world races to control the spread of COVID-19 and find a vaccine, what role can artificial intelligence play?
This is the question that Yoshua Bengio, founder of Mila and winner of the 2019 ACM A.M. Turing award, and his team are trying to answer.
Bengio and his collaborators have harnessed the potential of AI through two projects aimed at early detection and discovery of new antiviral drugs, he said in his Breakthrough Days keynote during the AI for Good Global Summit 2020.
Predicting viral load with machine learning
The first project, called COVI, is a peer-to-peer tracing app that uses AI to predict how infectious a user with COVID-19 is likely to be. The goal is to help them understand their risks and inform their behaviour.
The factors that inspired COVI are quite straightforward. “One of the big problems with COVID-19 is that a lot of the people are infecting others without knowing they have the virus,” said Bengio. The viral load, which is the quantity of virus inside a person, can be high even before they show any symptoms.
The COVI team felt that helping people understand how infectious they are would lead them to take appropriate safeguarding actions such as wearing a mask, social distancing or self-isolating at home. They could also accordingly send secure messages to inform those who have come into contact with them about their exposure and risks.
COVI contains information entered by users about their symptoms, their pre-existing conditions and results of COVID-19 tests. It also keeps track of their recent contact history. Machine learning helps predict the viral load by simulating different conditions to assess risk levels. This, in turn, is used to recommend behaviour that will protect the user and those around them.
An advantage of this framework is that it can predict contagiousness from both the present and the past, making COVI a potentially quicker detection and vigilance tool than manual contact tracing methods or binary tracing that rely only on test results.
AI based on machine learning requires data to solve problems. But getting the data can be challenging due to political and privacy concerns, said Bengio.
“If people are not sufficiently convinced and reassured about this ‘weird’ technology, they are not going to use it. And if they don’t use it, it’s not going to work,” he said. Bengio also acknowledged the concerns around privacy. With COVI, the phone will receive a notification “with just enough information” about a stranger’s symptoms to help the user decide whether they want to wear a mask or change their plans to dine out, for instance.
AI-driven drug discovery
The second project at Mila involves machine learning to discover antiviral drugs to fight COVID-19, using graph neural networks to explore combinations of existing drugs and trying to search for all possible drug-like molecules.
“What you want to predict is both the binding affinity of the drug to the protein but also whether the drug is synthesizable or toxic,” Bengio said. “You train different neural nets for each of these things. And then we can check how good those predictions are,” he added.
This project also uses machine learning to generate new drugs through a sequence of changes in the candidate molecule and through iterative learning from the data to further train the system.
Tackling complex challenges using AI is a collective mission to share knowledge and promote transparency to achieve social impact, Bengio reiterated.
“When we start thinking about AI for good, we really need to think about how we can change political economic systems around the world to make these powerful technologies work for the common good and work for those who need it the most rather than those who have the most power,” he said.
Collective well-being for social impact
IBM Research Director Dario Gil also raised the importance of building “communities of discovery” to complement accelerated scientific discoveries during his Breakthrough Days keynote session.
Gil helped set up the COVID-19 High-Performance Computing (HPC) Consortium in March, drawing members from across the industry, academia and federal governments. The idea arose when the IBM Summit supercomputer was used to winnow down a list of 8,000 small molecules that could bind to SARS-CoV-2’s spike protein, which allows coronavirus to enter human cells, to about 77.
“If that was possible, we thought about what could be done if we aggregate enough supercomputing capacity and match it to the best scientists all over the world and prioritize it so that could happen,” Gil said. The consortium now has over 85 research projects on bioinformatics, epidemiology and molecular modelling running concurrently on 600 petaflops of supercomputing processing power.
The social perspectives required to fight COVID-19 using AI have also been a key theme of the projects proposed during the Collective Pandemic Intelligence Breakthrough Track.
One project is a ‘global COVID policy simulator’ using AI to build personalized predictions on how data reacts to changes in policies, adding country specific variables for an optimal policy mix.
Another is a smartphone-based initiative which feeds self-reported symptoms, including breathing sounds, heart rate and GPS data to a machine learning system that combines these inputs with deep learning algorithms for a COVID-19 risk index. The third project proposed early detection infrastructure linked to wastewater-based epidemiology for vulnerable communities.
In addition to these promising Breakthrough Days projects, a new ad-hoc group led by ITU and the World Health Organization (WHO) aims to establish best practices in the use of AI during each phase in the lifecycle of a public health emergency. “These solutions will also play a role in the transition to living with COVID-19, in ongoing risk assessment, and in helping health services cope with the challenges of pulsed containment,” said Co-Chair of the ad-hoc group, Shan Xu of the China Academy of Information and Communications Technology.
The ad-hoc group forms part of the ITU-WHO Focus Group on Artificial Intelligence for Health, a multistakeholder effort working on a benchmarking framework for ‘AI for Health’ solutions, including testing the efficacy of algorithms, in order to make them safer and viable in the fight against potential future pandemics.
Image credit: Gustavo Fring via Pexels