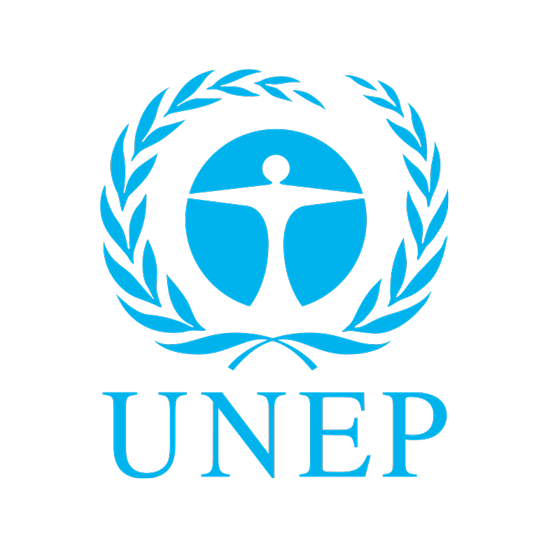
The United Nations Environment Programme (UNEP) is the leading global environmental authority that sets the global environmental agenda, promotes the coherent implementation of the environmental dimension of sustainable development within the United Nations system, and serves as an authoritative advocate for the global environment.
Description of Activities on AI
Project 1: Artificial Intelligence tool for valuing the contributions of nature (ARIES for SEEA)
The project aims to accelerate implementation of the new ground-breaking standard for valuing the contributions of nature that was adopted by the UN Statistical Commission in March 2021, the System of Environmental-Economic Accounting (SEEA) Ecosystem Accounting.
Artificial Intelligence for Environment & Sustainability (ARIES), developed by researchers at the Basque Centre for Climate Change (BC3), is an integrated, open-source modelling platform for environmental sustainability, where researchers from across the globe can add their own data and models to web-based repositories.
Using ARIES technology, the ARIES for SEEA Explorer application allows users anywhere in the world to produce rapid, standardized, scalable and customizable ecosystem accounts for their area of interest that are consistent with the SEEA Ecosystem Accounting framework. ARIES for SEEA is available on the UN Global Platform, a cloud-service platform supporting international collaboration in the development of official statistics using new data sources and innovative methods.
The ARIES for SEEA platform is currently being piloted in Botswana, Ghana, Uganda, Rwanda, Senegal and South Africa, following a workshop in Kigali in July 2022.
Project 2: Adaptation to climate change in sub-Saharan African humanitarian situations
The project harnesses artificial intelligence to investigate past environmental change around selected humanitarian hotspots of displacement in Burundi, Chad and Sudan and future projections in the face of the climate crisis to inform climate change adaptation measures and anticipatory action for integration in humanitarian programming.
Project 3: Identifying the potential applications of Artificial Intelligence for Disaster Management
Rapid advances in science and practices of Artificial Intelligence (AI), Robotics, Drones and Internet of Things (IoT) is expected to change all aspects of human society in the coming decades. Social scientists call this collective change the “Fourth Industrial Revolution” (4IR).
There are many possibilities for introducing these technologies into the domain of disaster response, especially for environmental disaster response. In addition to helping mitigate the consequences of disasters, the technologies of the fourth industrial revolution also help prepare for them.
The proposed project is aimed at the current state of play on the applications of 4IR technologies for disaster management and identifies opportunities and partners to promote these technologies for environmental emergency response in the future.
For instance, in October 2020, during the AI for Good Summit organized by ITU, UNEP led a session on Artificial Intelligence for Natural Disaster Management. In addition to UNEP and ITU, the session had speakers form Google, Government of India and Monash University. With over 2000 registrations, it was the most well attended session at the AI for Good summit. This led ITU, UNEP and WMO to form a partnership to take this work forward.
Project 4: Global Partnership on Marine Litter (GPML) Recommender
The Global Partnership on Marine Litter (GPML) Digital Platform is a multi-stakeholder platform that compiles different resources, connects stakeholders, and integrates data to guide action. Its vision is to be the go-to open source multistakeholder Platform that successfully connects and informs all actors working towards addressing the global problem of marine litter and plastic pollution.
The Platform allows stakeholders to get an overview of the different initiatives, find relevant resources and connect with other stakeholders in the field. To encourage and make it easier for stakeholders to connect, UNEP would like to implement a matchmaking system that automatically matches stakeholders based on their interests, skills, and other relevant information.
Project 5: Using Machine Learning to Make Government Spending Greener
The project seeks to show how machine learning (ML) models can help policy makers and researchers design data-driven policies that most efficiently and effectively allocate scarce government resources at home and abroad to maximize inclusive and sustainable prosperity and development.
For policy- and decision-makers in many countries, one of the key impediments to designing well-targeted green transition policies is a lack of data and intelligence on the causal chains from a policy to its impact on society, the economy, and the environment. It is difficult to manage and prioritize green spending if you can’t measure its e effects.
Properly trained machine learning (ML) models can enable rapid, quantitative predictions of policy impacts.
This exploratory research venture between the UN Environment Program (UNEP) and UNCTAD showcases how machine learning has the potential to transform the measurement of policy impacts on Sustainable Development Goals (SDGs), Nationally Determined Contributions (NDCs) and National Biodiversity Strategies and Action Plans (NBSAPs) and enable targeted and efficient decision making for underpinning green and inclusive transitions.
Illustrative models and analyses were created for 6 different countries: Zambia, Haiti, DRC, Solomon Islands, Liberia, Madagaskar
Project 6: SDG-meter
Textual documents produced and consumed by UN frequently require identifying its linkages to SDGs (project proposals, reports, briefings, etc). This mapping demands extensive expert time and rely on personal knowledge of interlinkages among topics and SDGs. While UNEP counts with experts in several topics, identification of SDGs outside their expertise can be missed out.
The SDG-Meter, a web platform based on one of the most sophisticated deep learning – natural language processing algorithms (BERT), permits to analyze text document via and rate relation to each of the 17 SDGs in percent.
Project 7: Predictive analytics to support governments in transforming food systems (TEEBAgriFood)
This project catalyzes the use of predictive analytical tools to support governments in transforming food systems faster, better and at a greater scale than before.
Predictive analytics and scenario modeling allow decision makers to compare future policy intervention options in the food system, illuminating the full impacts of a policy decision on natural, social, human and produced capital. This enables strategic foresight and anticipatory decision-making that values instead of discounts the future.
Modeling techniques and tools are increasingly becoming more efficient through machine learning and the use of Earth Observations. Models applied for such exercise can range from ‘snapshot’ biophysical ecosystem service models (such as InVEST) up to the modeling of dynamic biophysical and socio-economic systems including dynamic relationships between conflicting or compounding government policies (for example agriculture subsidies and environmental regulations).
Applications are initiated in Brazil, China, India, Indonesia, Mexico, Thailand, Malaysia, Colombia, Kenya, Tanzania, Thailand and Mexico.