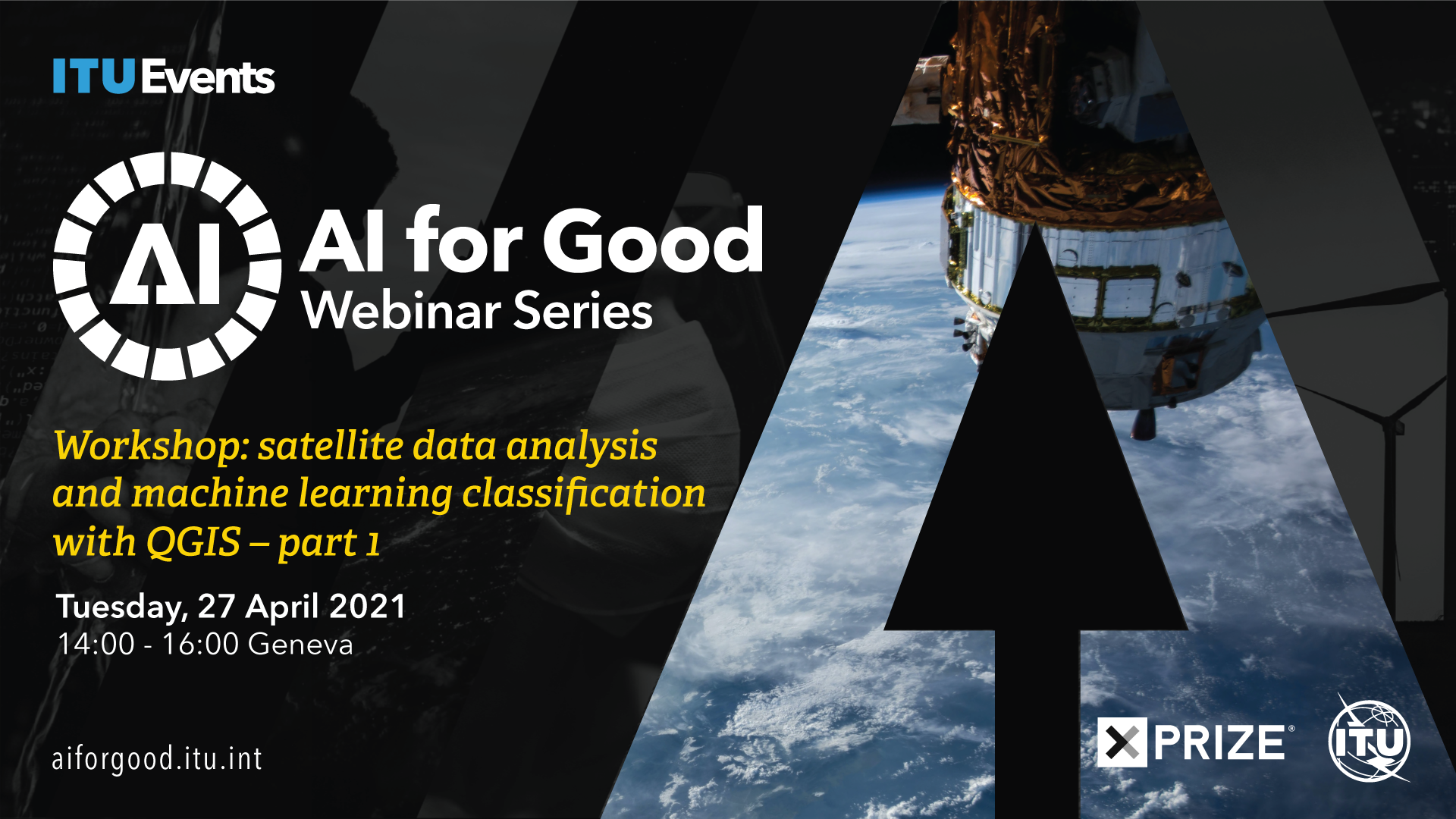
Would you like to increase your skills in the field of satellite images processing using Machine Learning? Do you know the basics of GIS (geographic information system) or QGIS (an open-source application to view, edit and analyze geospatial data)? Then please feel free to join this workshop! It introduces how classification of satellite imagery can be done with QGIS by showing how to retrieve, process and classify satellite imagery, as well as how to assess performance of machine learning algorithms through error matrix and accuracy indexes.
The workshop involves two QGIS plugins: Semi-automatic Classification Plugin (SCP) and dzetsaka. SCP is used for majority of preprocessing operations such as retrieval of the Sentinel 2 imagery for an area of interest, DOS (Dark object subtraction) atmospheric correction, selection of specific bands for classification, creation of composite and computation of band algebra (i.e., Normalized Difference Vegetation Index (NDVI). The dzetsaka plugin is used to detect and classify built-up areas starting from preprocessed satellite imagery with Gaussian Mixture Model, Random Forest and K-Nearest Neighbors machine learning algorithms.
Besides the two plugins, some core QGIS functionalities and are included in the workshop for clipping satellite imagery and creating vector file of training data. Lastly, outcomes of the machine learning algorithm are compared with the global map of human settlements – GHS-BUILT (Sentinel-1) produced by Joint Research Center (JRC) of European Commission to assess their performance. Before being used for assessing algorithms’ performances, GHS-BUILT (Sentinel-1) is adapted to coordinate reference system, resolution, and classes of classification outcomes. Adaptation of GHS-BUILT (Sentinel-1) involves many isolated operations (reprojection, tile merging, resampling, and reclassification). For this reason, the QGIS Graphical Modeler is introduced in the exercise because it allows automation of chain of operations. Besides the adaptation of GHS-BUILT (Sentinel-1), the computation of error matrix and accuracy indexes for each classification outcome are integrated with the Graphical Modeler too.
Please see the two one-pager guidelines for the software requirements and the datasets for the exercises and download relevant software and datasets before the workshop.
- Information and Requirements for Workshop
- Instructions for Attendees (Updated 7 May 2021)
- Now with two links to download the data (Google drive; Zenodo) and FAQs:
- Can I use a different version of QGIS?
- How can I find version 3.10. on the QGIS website?
- How to check if I successfully installed scikit-learn?
- Now with two links to download the data (Google drive; Zenodo) and FAQs:
- Slides set of Part 1, Satellite Data Analysis and Machine Learning Classification with QGIS (Posted 26 April 2021)
The workshop has two parts:
- Part 1: 27 April 2021, 14:00 – 16:00 CEST
- (Optional) 13:00 – 13:45 CEST Demo session on how to install the required software for the workshop on Windows OS.
- 14:00 – 16:00 CEST Workshop Part 1
- Part 2: 11 May 2021, 14:00 – 16:00 CEST