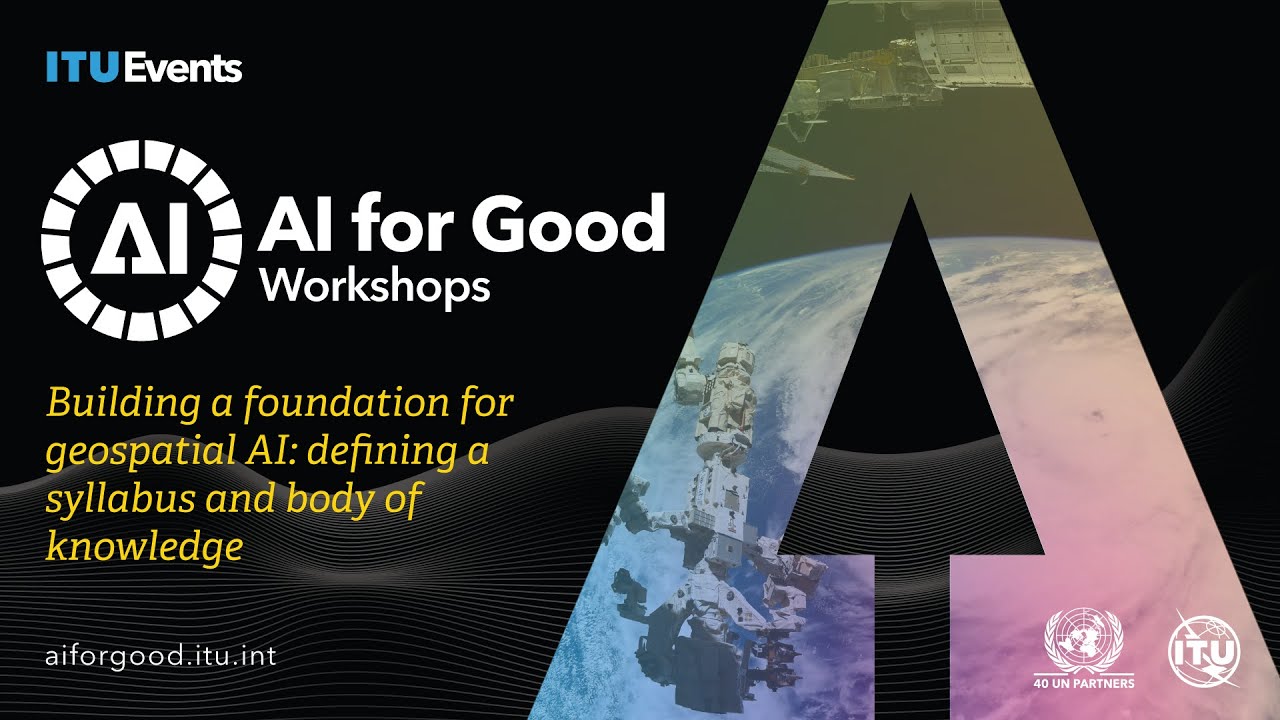
This is a replay of a workshop that took place in the ITU on the 5th of July, during the AI for Good Global Summit in Geneva, Switzerland.
Geospatial AI refers to the application of artificial intelligence (AI) methods, such as deep learning and machine learning, to process and analyze geospatial data. This allows us to better understand the physical world and our interactions with it at different levels, from individual to global. Geospatial data includes any information connected to a location on Earth, such as UAV, satellite, and sensor data, as well as maps, GPS tracks, socioeconomic data from Census, transaction data and textual references to locations like in restaurant reviews and Tweets. AI can extract insights and patterns from geospatial data that traditional techniques may not be able to find.
As geospatial experts, we can make a twofold contribution. In geodata-driven AI, machine learning models are trained on large datasets of geodata to discover patterns and relationships. Our experience in geospatial data is essential in teaching where to find, sample, collect, clean, and validate geodata. This helps to avoid the effect of “garbage in, garbage out.” In model-driven AI, explicit models of the problem domain are designed based on a deep understanding of the underlying principles and relationships. In geomatics, these models are typically based on mathematical or physical equations from various problem-domain specific disciplines which need to be enriched with AI methods and models while incorporating interdisciplinary perspectives.
Although the importance of integrating geospatial information and artificial intelligence is widely recognized, there is currently no shared syllabus or body of knowledge within the geospatial sector. Geospatial AI and data science solutions often use ideas and models from multiple disciplines. While one can’t be an expert in all relevant areas, a foundational understanding of different perspectives is really valuable for the geosal AI practitioners. Therefore, defining a syllabus is urgently needed to provide structure, clarify expectations, and help with planning in courses related to geospatial AI. A syllabus also helps to highlight the importance of geospatial AI and the relevance of geospatial data in this emerging field.
On the other hand, providing a body of knowledge in geospatial AI is crucial to establish a common language and understanding of key concepts, principles, and techniques. This promotes effective communication and collaboration among researchers, practitioners, and stakeholders in this field. It also promotes best practices in the collection, analysis, and interpretation of geospatial data and their integration with AI techniques. The curriculum should be an integrative framework to prepare next-generation of students and GeoAI professionals to tackle complex challenges that can only be solved with a holistic and interdisciplinary approach like GeoAI. Such a body of knowledge would enable further innovation and creativity in the development of new techniques, tools, and applications, leading to more breakthroughs in this field. Most importantly, it supports education and training programs by providing a framework for curriculum development and assessment. Finally, a body of knowledge facilitates decision-making in policy, planning, and management, allowing decision-makers to use geospatial data and AI techniques to address complex social, economic, and environmental challenges in a more effective and efficient manner.