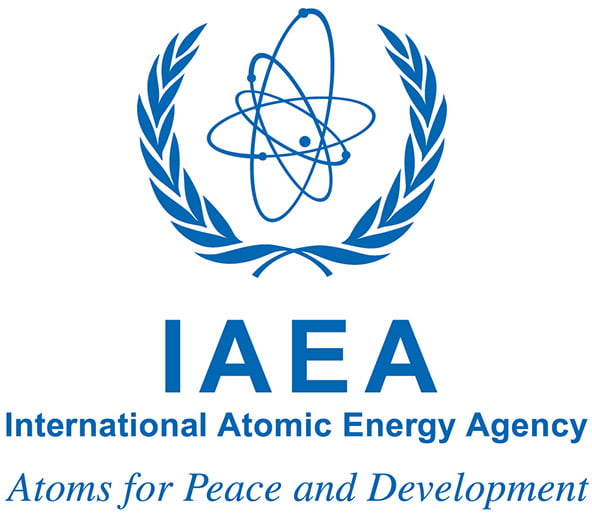
The International Atomic Energy Agency is the world’s central intergovernmental forum for scientific and technical co-operation in the nuclear field. It works for the safe, secure and peaceful uses of nuclear science and technology, contributing to international peace and security and the United Nations’ Sustainable Development Goals.
Description of Activities on AI
Project 1: Artificial Intelligence for Accelerating Nuclear Applications, Science and Technology, Non-serial Publications, IAEA, Vienna (2022)
This publication provides a review of the current state of the art, and outlines challenges and identifies opportunities for accelerating nuclear applications, science and technology with AI. After the introduction and background information on AI and its use in the nuclear field, Chapter 2 provides a summary of the ethics of AI and nuclear technologies and of the use of AI in nuclear applications, science, power, radiation protection, security and safeguards verification.
State of the art, priorities for future AI activities in the nuclear field and the IAEA’s role to support their implementation are presented in the following Chapters. Chapter 3 addresses the ethical impact of the convergence of AI and nuclear technologies. Chapters 4–12 focus on the applications of AI in nuclear fields corresponding to IAEA’s areas of work, including human health, food and agriculture, water and environment, nuclear data, nuclear physics, fusion, nuclear power, nuclear security and safeguards verification.
Project 2: AI for Atoms – the IAEA’s knowledge-sharing platform for partnership on AI applications in the nuclear field
The IAEA provides interdisciplinary fora for professionals to discuss and foster collaboration on the use of AI in nuclear applications, science and technology and is committed to sharing knowledge and forging partnerships through its AI for Atoms platform. As part of this initiative, the IAEA cooperates with the International Telecommunication Union, the UN Interagency Working Group on AI and almost 40 other UN organizations to provide a solid foundation for accelerated sustainable development with AI. The AI for Atoms platform provides information on the IAEA’s activities on AI, featuring all related initiatives, news, publications and events.
Project 3: Ethics of Nuclear and AI Applications
With its potential to accelerate reaching the Sustainable Development Goals by 2030, the use of AI can also benefit the peaceful uses and applications of nuclear technology in different sectors, including human health, food production, water management and environmental protection.
However, the convergence of AI and nuclear technologies could exacerbate existing ethical concerns in the disciplines as well as give rise to new concerns at their interface. Because both disciplines deal with risk and uncertainty and hold huge potential for both benefit and possible harm, there is a need for promoting awareness among practitioners about the ethical impact of the convergence of AI and nuclear science, technology, and applications, while creating mechanisms for robust dialogue with stakeholders.
This project intends to explore path forward to an international and multi-stakeholder cooperation aimed at societally accepted and ethically informed decision-making on the use of AI tailored for the nuclear field.
Project 4: AI for Fusion – accelerating fusion R&D with AI, through the creation of a platform and cross-community network for innovation and partnership
The IAEA has launched a 5-years Coordinated Research Project (CRP) on AI for Accelerating Fusion R&D (2022–2027) with the objective of accelerating fusion R&D with machine learning and AI, through the creation of a platform and cross-community network for innovation and partnership.
Project 5: AI to Assess Climate Impact on Global Lakes
Global warming is considered a major threat to Earth’s lakes water budgets and quality. However, flow regulation, over-exploitation, lack of hydrological data, and disparate evaluation methods hamper comparative global estimates of lake vulnerability to evaporation. The stable isotope composition of 1,257 global lakes was analyzed using Artificial Intelligence techniques. It was found that in most of the lakes, this depends on precipitation and groundwater recharge subsequently altered by catchment and lake evaporation processes. Isotope mass-balance modelling shows that ca. 20 % of water inflow in global lakes is lost through evaporation and ca. 10 % of lakes in arid and temperate zones experience extreme evaporative losses >40 % of the total inflow. Precipitation amount, limpidity, wind speed, relative humidity, and solar radiation are predominant controls on lake isotope composition and evaporation, regardless of the climatic zone. The promotion of systematic global isotopic monitoring of Earth’s lakes provides a direct and comparative approach to detect the impacts of climatic and catchment-scale changes on water-balance and evaporation trends. This project aims to establish a global network of isotopes in lakes (GNIL) to improve our AI-based assessments. Current activities include the design of the database, the selection of variables and the assessment of global coverage. Stable water isotope assays provide a low-cost effective tool to study lake-catchment changes with regards to sample collection and analysis. Additionally, stable isotope data from lakes is fully comparative globally, thereby providing a competitive advantage under the current scenario of different international methods and approaches that are not easy to compare in time and scale and which result in the current lack of the comparable data for lakes and catchments.
Project 6: Working Group on AI for Water and Environment
The Working Group (WG) on AI for Water and Environment – established in connection with the IAEA Technical Meeting on AI for Nuclear Technology and Application (2021) – aimed to promote and enable the use of isotopic techniques with AI tools for better management of water and environmental resources, as well as adaptation to climate change worldwide. Recognizing that with the increasing availability of data from satellites, unmanned airborne vehicles and sensor networks, there is a myriad of data available to couple and explore in conjunction with the IAEA’s global isotope databases.
The WG is a platform for scientists working with AI tools at the interface of isotope hydrology, water resources protection and management. This facilitates sharing of experiences in the use of machine and deep learning for hydrological and environmental modelling, challenges, and research opportunities to move forward. The WG aims to find synergies between isotope techniques, high-frequency or remote sensing, open-source resources, and AI to show how these can help inform policies to mitigate the world’s water problems. Besides exchanging knowledge on current developments in the area that feed into research activities of the isotope hydrology section, the WG is working on a review paper to be submitted to a hydrological journal that showcases different applications of AI in isotope hydrology. In this paper, the WG intends to explore what role machine learning and big data can play in the advancement of isotope hydrology.
Project 7: AI Assistance for Non-Destructive Testing in Disaster Management
Over the past few decades, the world has witnessed many natural and man-made disasters such as earthquakes and explosions with varying degrees of destruction and attendant loss of lives. The enormous effect of these disasters on civil engineering structures constitutes the impetus for the deployment of Non-Destructive Testing (NDT) in the mitigation, preparedness, response and recovery stages of the disaster management cycle. Recognising the potential of AI to assist NDT by improving testing accuracy, speed of both inspection and data processing, and reliability and facilitate the interpretation of disaster management results coupled with the need for the NDT emergency response centre to assist IAEA Member States in the event of a disaster, a Technical Meeting is being planned to bring together experts in this field to discuss and define areas of focus for action.
Project 8: Working Group on AI for Human Health
The Working Group on AI for Human Health (WG-AI4HH) – established in connection with the IAEA Technical Meeting on AI for Nuclear Technology and Applications– focuses on possible approaches to the use of AI in specific human health domains. The WG-AI4HH is working on identifying the current and future support that should be provided to Member States in the field of AI applied to radiation oncology, nuclear medicine, medical imaging, medical physics and nuclear nutrition assessment. The potential and challenges of AI are investigated to ensure an informed, safe, ethically responsible and meaningful use of AI-based tools in the clinical environment. The working group is aware that quality of data and their curation is also fundamental to obtain reliable AI applications. Furthermore, the WG-AI4HH is monitoring trends of AI in health education to be eventually considered for education/training activities in the future. Furthermore, a new IAEA publication describing roles and responsibilities of, as well as educational and training needs for medical physicists using AI is being prepared.
Project 9: AI-assisted Contouring Skills in Radiotherapy
The IAEA is conducting a Coordinated Research Project on The Potential of E-learning Interventions for AI-assisted Contouring Skills in Radiotherapy (CRP E33046). The objective of the project is to investigate changes in inter-observer variation and bias after e-learning in delineation guidelines and the use of deep learning-based auto-segmentation of organs-at-risk in head-and-neck cancer. In recent years, AI-algorithms, namely deep learning-based algorithms, have improved auto-segmentation drastically. It is believed that AI-tools lower variation and increase the accuracy and compliance of plans, which improves the chance of cure. AI-tools may also make care more cost effective by reducing the human capacity required, which is important in our overstretched health systems. A wide palette of commercial deep learning-based auto-segmentation solutions are emerging with the promise of leveraging the aforementioned benefits. The selection and contouring of target volumes and organs-at-risk has become a key step in modern radiation oncology. Concepts and terms for definition of gross tumour volume, clinical target volume and organs at risk have been continuously evolving (e.g., through International Commission on Radiation Units and Measurements (ICRU) reports 50, 62, 78, 83) and have become widely disseminated and accepted by the European and international radiation oncology community. 102 participants from 23 IAEA Member States from 24 radiotherapy centers are taking part in the project.
Project 10: AI to Support Remediation of Radioactive Contamination in Agriculture
Remediation of radioactive contamination of farmland requires accurate soil data. In case of nuclear emergencies affecting food and agriculture, exchangeable potassium (Kex.) plays a major role. As potassium competes with radiocaesium in soil-to-plant transfer, it can help reduce the crop uptake of this major fallout radionuclide. Information on potassium content in the soil is essential for optimizing remediation of radioactive contamination. Through the international research network under the IAEA funded Coordinated Research Project D1.50.19 on ‘“Remediation of Radioactive Contaminated Agricultural Land”, the Joint FAO/IAEA Centre of Nuclear Techniques in Food and Agriculture investigated how to predict exchangeable potassium in soil through Mid-Infrared Spectroscopy and Artificial Intelligence. In our study, which was finalized this year and is expected to be published soon, we show how this can be now achieved using a Convolutional Neural Network (CNN) model trained on a large Mid-Infrared (MIR) soil spectral library (40000 samples Kex determined with 1M NH4OAc, pH 7), compiled by the National Soil Survey Center of the United States Department of Agriculture. Using Partial Least Squares Regression as a baseline, we found that our implemented CNN leads to a significantly higher prediction performance of Kex. when a large amount of data is available (10000), increasing the coefficient of determination from 0.64 to 0.79, and reducing the Mean Absolute Percentage Error from 135% to 31%. Furthermore, in order to provide end-users with required interpretive keys, we implemented the GradientShap algorithm to identify the spectral regions considered important by the model for predicting Kex. Used in the context of the implemented CNN on various Soil Taxonomy Orders, it allowed (i) to relate the important spectral features to domain knowledge and (ii) to demonstrate that including all Soil Taxonomy Orders in CNN-based modeling is beneficial as spectral features learned can be reused across different, sometimes underrepresented soil orders.
Project 11: AI to Assess and Use Marine Environmental Radioactivity
Levels of radionuclides in the marine environment can be influenced by a range of manmade inputs (e.g., nuclear accidents and authorised discharges from nuclear fuel cycle facilities), underlying biogeochemical conditions, and other dynamic such as climate change and sea level rise. On the other hand, the presence of radionuclides – both natural and artificial – in the marine environment offers a wealth of possibilities for the application of radiotracers for quantification of climate and ocean change. The IAEA Marine Radioactivity Information System (MARIS) has been maintained for many years to provide data underpinning related monitoring and research activities. MARIS is currently being redeveloped in order to offer improved support and possibilities to its user community, including for AI and ML applications. The planned improvements include adoption of Open Science principles, data management according to FAIR and/or GEO principles, improved API access and adoption of netCDF and related technologies, with all associated improvements in metadata provision and opening of access to a rich range of tools for accessing, analysing and visualizing data. The overall aim is to promote data re-use and to facilitate Open Science. Specifically for AI we foresee a range of possibilities incorporating marine modelling for prediction of inter alia transport, dispersion and settling rates for future and sparsely observed scenarios; visualisation of radionuclide levels in four dimensions; time series analysis; improved radiotracer applications and reanalyses using combined datasets.
Project 12: Working Group on AI for Nuclear Power
The Working Group on AI for Nuclear Power – established in connection with the IAEA Technical Meeting on AI for Nuclear Technology and Application (2021) – aims at discussing the potential applications of AI, as well as the main opportunities for AI to have positive impact on the nuclear power industry. A wide range of applications of AI and machine learning were summarized in the technical report from the AI nuclear power working group meeting, held in October 2021. The advancements and applications in AI are expected across the spectrum of nuclear power project lifecycle, including R&D, engineering, construction, operation and maintenance (O&M) and decommissioning.
During a Consultancy Meeting (2022), members of the Working Group and other experts, including representatives from regulators from two IAEA Member States, discussed the utilization of AI in nuclear power plants (NPPs) in operation today as well as designs being considered in the future. Focusing on operating plants, it emphasizes the desired context of existing design control, O&M, regulatory frameworks, processes and procedures. The aim would be to understand technological challenges, knowledge gaps, and resources required via collaborative effort among Member States, enabling development, demonstration, and application of explainable artificial intelligence (XAI) technologies in the current and future nuclear reactors, advancing the level of autonomy and plant modernization.
Several challenges with respect to deployment were also identified. Observations associated with technological challenges, knowledge gaps, and resources requirements were collected for IAEA with the objective of accelerating progress, including both R&D phase as well as the transition from R&D to deployment. Based on these observations, a set of recommendations are developed as path forward. The working group serves as an effective platform to share these ideas and put forth the vision for possible activities in the area of AI for nuclear power.
A Technical Meeting on AI and Its Recent and Near-term Deployment in Operating NPPs is being planned for 16–19 May 2023, Vienna, Austria, with the purpose of gathering and sharing Member States experience related to plans for near-term deployment of AI solutions in operating NPPs. The event is also expected to gather experience from solutions already being used at operating plants in the nuclear power sector.
Project 13: IAEA Technical Meeting on the Safety Implications of the Use of AI in Nuclear Power Plant
The purpose of the event is to collect current experiences in Member States on the use and application of AI techniques to support different aspects of the design, safety assessment and operation of Nuclear Power Plants; and to understand challenges related to safety demonstration and justification of AI applications. Ultimately, the information will be used to plan future work to develop and/or update IAEA guidance on AI.
Project 14: Working Group on AI for Nuclear Security
A Working Group (WG) on Artificial Intelligence for Nuclear Security (AI4NS) – established in connection with the IAEA Technical Meeting on AI for Nuclear Technology and Application (2021) – discussed AI across different areas of nuclear security, including cyber and information security, forensics, detection, material security, and insider threats. During the WG sessions, 16 IAEA Member State experts led discussions on present uses and future opportunities for AI and related technology for nuclear security. The experts shared challenges, observations, and lessons learned in developing, using, and regulating AI technology; and discussed potential risks introduced or reintroduced by the use of AI techniques and technology. The need for more collaboration, investigation, and information exchange on the positive and negative impact and implications of AI in nuclear security was identified.
The WG on AI4NS sessions were dedicated to the following thematic areas: anomaly detection; data analysis (flow, sensor, image); data integration; data management; defensive computer security (network) architecture; internet of things – cloud services; information protection; performance assessment; systems design analysis; threat analysis; training; vulnerability management; and adversarial AI.
Project 15: AI-Assisted Processing of Safeguards-relevant Information
The collection and evaluation of all safeguards-relevant information is one of the fundamental processes of safeguards implementations for the IAEA. The rapid increase in availability of large amounts of data and media types calls for new approaches to assist analysts in spotting “the signal in the noise”.
Project 16: Working Group on AI for Safeguards Verification
The Working Group on Artificial Intelligence for Safeguards Verification – established in connection with the IAEA Technical Meeting on Artificial Intelligence (AI) for Nuclear Technology and Application – focused on two different applications of AI in safeguards activities: verification of spent fuel and video surveillance. Using AI for spent fuel verification is extremely relevant to safeguards due to growing inventory of fissile material. Gamma spectroscopy and Cerenkov imaging data are utilized and numerical simulations supply training and test datasets for the models. These AI algorithms are interpretable and can be justified with physics. The accuracy for AI methods in spent fuel verification is sometimes on par with traditional instruments; however, the technology is not yet mature enough to make autonomous decisions.
Implementing AI for video surveillance could allow for large productivity gains in safeguards. Surveillance is challenging and time-consuming and AI could help with these issues. Data is acquired from similar facilities under surveillance and from simulations/digital twins. Algorithms can be used for many different applications including object detection, object tracking, anomaly detection, and processing of open-source data. Because of the consequences of missed events, improvements are needed to penalize false negatives.