Probing Earthquake Faults with AI
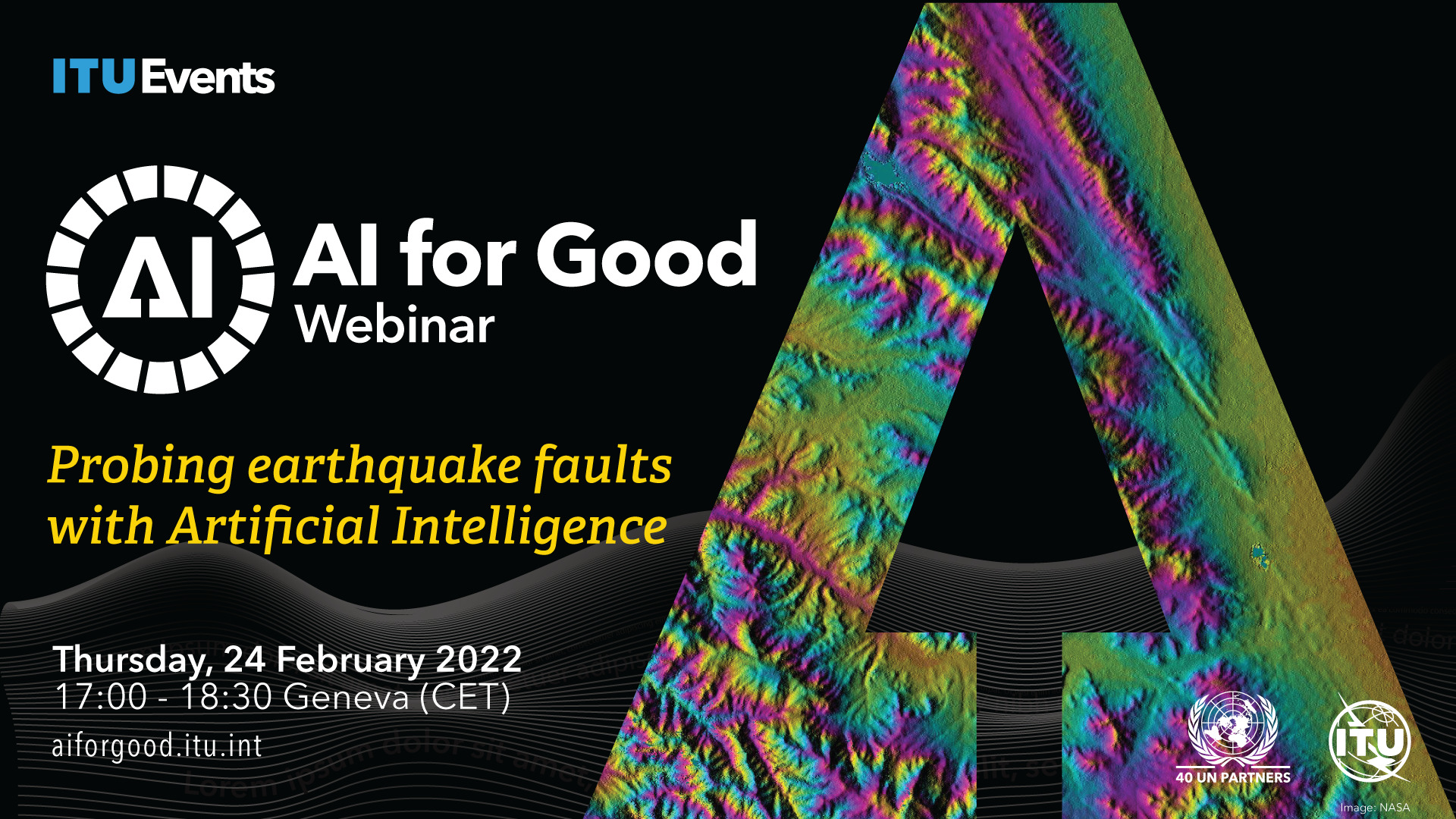
* Register (or log in) to the AI4G Neural Network to add this session to your agenda or watch the replay
Ask a geophysicist whether earthquakes can be predicted, and they will answer with a resounding “no”. Determining the location, magnitude, and timing of an upcoming earthquake is extremely challenging and some believe impossible. Attempts so far over the last 50+ years have failed; however, recent work applying AI methods applying geophysical data streams provides a glimmer of hope toward characterizing fault physics and forecasting earthquake timing.
Machine learning methods require lots of training data. But data simply doesn’t exist for an entire earthquake cycle, i.e. the time where stress on a fault (such as the San Andreas fault in California) builds up over a long period of time (say, years or decades) until the stress is suddenly released in an earthquake. Geophysical data exist usually only for a portion of an earthquake cycle. This makes AI very challenging for earthquake studies, so how to proceed?
The approach that we settled on was to apply laboratory earthquake experimental (‘labquake’) data to develop AI skills that could ultimately be applied in Earth. Labquakes are miniature earthquakes generated on scaled-down faults in a laboratory located at the Pennsylvania State University and operated by Chris Marone. Simultaneously, we began conducting computer simulations of lab-scale quakes. We found that machine learning models that have been trained on lab quake, acoustic emission (AE) data do a surprisingly good job in predicting fault properties such as the fault friction and the fault displacement, as well as the timing of upcoming lab quakes. Remarkably, the AE contains a fingerprint of the fault behavior at all times. But these models still require much training data, far too much to be applied in Earth except under certain circumstances.
Slow-slip events (also called silent earthquakes) are different from earthquakes in that a slow-slip event releases its energy not in seconds like in an earthquake but over much longer periods of time – in hours, days, or even months. Thanks to these elongated timescales, many slow earthquakes are available to train a machine learning model. Geophysicists have measured slow-slip events along a 1000 km stretch of the coast of North America over the last three decades. Our team was able to “hindcast” slow-slip event timing based on the data which preceded them to about within a week.
Following this work, we devised a path forward for seismogenic faults—those that cause earthquakes—using the concept of transfer learning. Computer simulations of faults are relatively inexpensive to conduct for many fault cycles. We found that when the data from the computer simulations are enriched with data from an actual lab experiment, predictions are very good. This concept, building computer simulations of a fault in Earth and enriching the model with a small portion of actual data from the fault is a path forward to Earth. Simultaneously, we are intending to include a model of frictional behavior in the deep learning approach we are developing. As we develop and apply these approaches to seismogenic faults, we will determine whether progress can be made on the challenging prediction problem in Earth.
This live event includes a 30-minute networking event hosted on the AI for Good Neural Network. This is your opportunity to ask questions, interact with the panelists and participants and build connections with the AI for Good community.