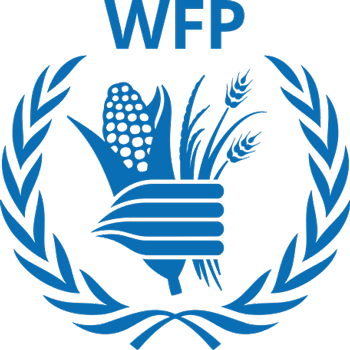
The World Food Programme is the world’s largest humanitarian organization, saving lives in emergencies and using food assistance to build a pathway to peace, stability and prosperity for people recovering from conflict, disasters and the impact of climate change.
Description of Activities on AI
Project 1: SKAI
A lack of on-the-ground information at the start of a humanitarian crisis is a major obstacle to a quick, effective response. Following disasters, WFP works to assess the magnitude of damage, the needs of local communities, and its humanitarian intervention plans to mobilize resources and coordinate emergency response efficiently. As part of its frontier innovations portfolio, WFP has been exploring new technologies that can automate this process and speed up response times. WFP partnered with Google Research to set up SKAI, a humanitarian response mapping project powered by artificial intelligence — an approach that combines statistical methods, data and modern computing techniques to automate specific tasks. SKAI assesses damage to buildings by applying computer vision — computer algorithms that can interpret information extracted from visual materials such as, in this case, satellite images of areas impacted by conflict, climate events, or other disasters. The key to this process is a machine learning model developed specifically for SKA, which detects damaged buildings by comparing imagery of the same buildings before and after the disaster. SKAI aims to leverage the power of artificial intelligence and remote sensing to assess damage within 24 hours after disasters take place.
Project 2: HungerMap LIVE
Understanding the food security situation requires a thorough analysis of data that are scattered across different data sources and platforms. HungerMapLIVE brings together different streams of publicly available information on food security, nutrition, conflict, weather and a variety of macro-economic data – including from WFP – all in one place to provide a holistic overview of the food security situation at global, country and sub-national levels. The resulting analysis is displayed on an interactive map using advanced data visualization tools.
As WFP’s global food security monitoring system, the HungerMap LIVE:
enhances operational effectiveness by identifying areas that are sliding towards food insecurity, providing information on shocks, hazards and other drivers of hunger in real-time, ensuring more informed and timely response to food crises;
maximises efficiency by providing continuously updated data at a lower cost and in less time, compared to traditional food security monitoring systems alone;
ensures continuously updated information in countries of interest, regardless of accessibility issues and the scale of WFP’s operational presence.
Project 3: MEZA (an Optical Character Recognition system that uses Artificial Intelligence to digitize handwritten records, speeding up data collection and analysis processes and allowing decision makers make data-based decisions in a timely manner.
Nutrition records for millions of malnourished children lie in remote health clinics around the world. Many of these clinics record patient data using paper-based booklets, which may be easily lost or destroyed. Different stakeholders involved in the fight against malnutrition in affected countries acknowledge that the digitization of these conventional paper-based systems would increase the efficiency and effectiveness of malnutrition management efforts.
Meza is a tool powered by artificial intelligence, developed by Charitable Analytics International to help digitize handwritten data from the deep field. MEZA uses an Optical Character Recognition technology to rapidly collect nutrition and related health data from remote, low-resource health clinics, enabling WFP and governments to have the information they need to provide high-quality, context-specific, and timely nutrition support. Following a WFP Innovation Bootcamp in June 2018, WFP’s Nutrition Division of WFP and WFP’s Country Office in the Republic of Congo identified Meza as a potential solution that could enhance the digitization of beneficiary management systems in the Republic of Congo.
From November 2018 to April 2020, the tool was tested across 45 clinics supported by WFP in the Republic of Congo through two pilots funded by the WFP Innovation Accelerator.
Project 4: Optimus
WFP staff face complex, cross-functional challenges every day, often with many possible solutions. Whether it’s funding shortfalls, access restrictions due to rainy seasons, new import regulations, or operational scale-ups, every day it’s something new. To properly manage the complexity and to enable an agile comparison of potential alternatives, it is critical to use data and optimization to find the right solutions. Optimus is a web application that looks at WFP operations end-to-end to support better planning, helping users identify the most cost-effective solutions using advanced mathematics. It pulls together a wide variety of data—from beneficiary numbers to sourcing options and from transport routes to nutritional values. Users can create their own scenarios or ask Optimus to find optimal plans, taking into account operational restrictions such as lead times and funding and preferences such as nutritional value targets and local procurement targets. A user-friendly interface allows users from any functional area to quickly explore and compare different scenarios.
Project 5: Humanitarian Chatbots
In emergency and development contexts, communication with affected communities is crucial. Having access to accurate, tailored information and engaging in a dialogue contributes to the resilience and ability of people to cope with a crisis. Since 2016, WFP has been working on the development and rollout of humanitarian chatbots to help deliver vital information to the people in urgent need. This technology improves WFP’s outreach to populations in hard-to-reach areas using a mobile device or a computer and complementing existing communication channels and WFP’s food security monitoring systems. This technology has proven to be particularly useful during COVID-19, as communication with affected communities has become even more crucial in times of unprecedented uncertainty.
Chatbots are highly customizable. For example, chatbots can be deployed as part of an assets creation programme to provide information about the use of assets as well as to collect feedback from the users. As part of Complaint Feedback and Mechanism (CFM) systems, chatbots have been useful in offering access to information 24/7. Moreover, the analytics produced by chatbots can inform further interactions; WFP staffs can monitor errors and improve the technology and user experience over time. As such, chatbots are highly user-friendly and do not require a high level of computer literacy, making them accessible to large segments of the population.
Project 6: Voice-to-text AI phone survey tool
WFP’s field numerators may not be able to conduct face-to-face household nutrition surveys due to the COVID-19 restrictions. In addition, it is costly to conduct surveys through alternative means like call centres. We wish to use an artificial intelligence-powered IVR solution to automate the beneficiary surveying process. The project is set up to (1) test the commercially viable IVR service embedded with AI-powered speech recognition technology; (2) fine-tune open-sourced speech recognition models using audio training data from populations that best represent the demography of WFP beneficiaries in terms of age, gender, region, accent, dining behaviours, and etc.; and (3) compare the performance of the two technologies. Shall the technologies be proven effective, the project will aim for a corporate-wide adoption before scaling to the wider humanitarian and development sector.
Project 7: Child Growth Monitor
Malnutrition is a global health crisis and the leading cause of death among children under five. To be able to efficiently treat malnutrition, it must first be detected. This detection requires anthropometric measurements of weight, height, and middle-upper arm circumference. However, measuring children accurately is a challenge, especially in the Global South, due to limited resources, unreliable, bulky traditional measurement hardware and/or lack of trained enumerators. Child Growth Monitor (CGM), an AI-powered smartphone app launched by the German non-profit organization Welthungerhilfe, was created to overcome these issues. With CGM, we aim at developing a non-profit, open-source, mobile solution that leverages artificial intelligence, especially computer vision machine learning technology, to enable frontline-healthcare workers to quickly and accurately measure children under five years, using a smartphone. Our measurements will be used to support the diagnosis of malnutrition of children according to the WHO growth standards and provide quick and accurate data in the most ethical way to organizations working on the UN SDGs and in public health.
Project 8: Combination of Drone and Sentinel-2 data for crop type mapping over areas of resilience interventions
Crop land and type mapping is crucial in the assessment of agricultural production of a country. It is also a critical prerequisite for the monitoring and assessment of changes in agricultural livelihood resources, which is particularly important in areas affected by conflict, natural disasters and other disruptions, allowing staff to timely allocate resources and deliver food assistance. However, the collection of ground data tends to take considerable time and may be particularly expensive and hard to carry out in emergency contexts.
The current project explores innovative methodologies for making the crop type mapping process more efficient and cost-effective. A combination of images from drones and satellites such as Sentinel-2 open up new possibilities for obtaining accurate data from the ground. WFP is testing the use of drones to capture images from much larger areas, compared to conventional data collection methods which use enumerators or smartphone applications which lead to relatively small sample sizes. The drone images are then classified into different crop types with high or sufficient accuracy. The ultimate goal is to use the drone data to train the Sentinel-2’s machine learning model to recognise different types of crops over larger unsampled areas. As a result, this new process combining artificial intelligence with drone and satellite imagery data will substantially reduce field work and automate the process of crop type mapping.
Project 9: AI4Human – AI for Humanitarian Applications (DLRR and WFP)
Project 9A: AI for Human
AI for Human is an artificial-intelligence-assisted building damage detection and classification tool used in the aftermath of natural disasters such as floods, cyclones and earthquakes. The project was focused on the development of methods and processing environments to detect changes in infrastructure and buildings by using machine learning techniques. The main focus is on the demand-driven development of existing deep learning methods and the provision of software for humanitarian organisations, to speed up response times in emergencies. WFP is particularly interested in exploring and testing the use of artificial intelligence-enabled procedures, for instance,for detecting infrastructure damages caused by natural disasters. WFP will provide the datasets used for the case study The project will also evaluate and illustrate the opportunities and and limitations pertaining to the machine learning methods in this specific technology.
Project 9B: Fusion of Remote Sensing and Web-based data sources
This project explores the systematic development and evaluation of national and global data and metadata published on the Internet to assess its usability for the derivation of crisis-related information. National and global databases published on the Internet and blogs, newsfeeds and social media contributions are a complementary source of information on satellite imagery and derived products. Therefore, the acquisition and aggregation of such data and the integrated presentation of these two sources of information (e.g. in mapping products of the ZKI or web-based services) can greatly contribute to an overall analysis. The fusion techniques will be developed and tested grounded on past or ongoing crisis situations. For example, humanitarian emergencies such as floods in Mozambique in 2019 can be included in the model (from M1). Data on refugee camps and their dynamic developments will also be added systematically (from M13). Finally, analyses of food security issues such as post harvest loss estimation in Africa can also potentially enhance the model.
Project 10: Fampred
FamPred project is complementary to WFP’s HungerMap LIVE humanitarian mapping project (https://hungermap.wfp.org). FamPred expands the HungerMap LIVE’s capabilities to forecast food crises by adding projections on insufficient food consumption. Artificial intelligence offers new opportunities for forecasting food insecurity in complex systems, where an approach known as “reservoir computing” is among the most promising. The project aims to develop a reservoir-computing-based prediction model using relevant datasets for the identified geographies in HungerMap LIVE. This model would strengthen WFP’s and national governments’ capacities to predict crises, deploy resources and prevent food crises outbreaks, ensuring communities at risk of hunger continue to get the support they need, when they need it.