How can we solve the problems of gender bias in AI? Experts weigh in.
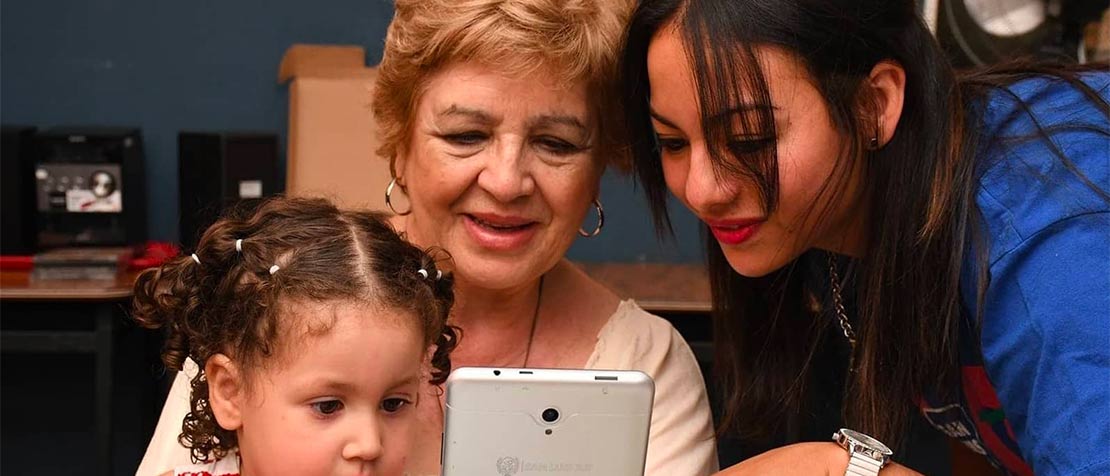
Artificial intelligence (AI) has a bias problem.
Actually, AI has many well-documented bias problems. Chief among these, arguably, is gender bias.
From the creation of data sets, to the way the data is collected and used, to the building of AI solutions – women are underrepresented at every stage. This means that AI solutions will not serve the needs of half the world.
If AI is to help accelerate progress on humanity’s greatest challenges, we will need to work together to solve AI’s many gender bias problems.
But how?
What equitable policies need to be made around AI? Why is diversity so important for data collection? What does algorithmic gender bias look like? How can AI show us COVID-19’s impact on roles in the workplace?
A group of experts gathered at yesterday’s opening ‘Breakthrough’ session of the annual AI for Good Global Summit to tackle these questions and more. (Due to the global COVID-19 pandemic, this year’s Summit is all online and will take place throughout the year.)
Including women at every stage
A large part of the problem is that the AI solutions are not being made with women in mind, agreed the panel during webinar.
“When you think about making something that is truly valuable for people, you have to think about how it’s helpful for every individual,” said Ida Tin, CEO and Co-Founder of Clue, a customized female health period-tracking app with 12 million active users worldwide.
“The problem with lack of diversity is that you have blind sides,” said Ms. Tin. “There is a profound blindness in the world that … is embedded in culture.”
Men often want to understand the issues women face, she said, but how would they know what those are? How would they even think to ask?
“A huge part of our need space was missed, because those who built products weren’t women” said Ms. Tin. “We desperately need diverse teams asking these questions. It’s fundamental. If not, you end up building a world that’s not for everybody.”
The panelists agreed that the stakes are high with AI and that the mistakes of the past must not be repeated.
“Why would the people you’re building solutions for not be involved in the process?” asked Kishau Rogers, CEO of Time Study, a startup offering solutions for using machine learning, advanced natural language processing, and data science to automatically tell a story of how enterprise employees spend their time.
“We are well beyond the time where we have to include the people that we are building the solutions for,” she said.
How does gender inequality in AI begin?
“Everything begins with data sets,” said Kaitlin Kraft-Buchman, Founder and CEO of Women@TheTable. “Data sets have left out women.”
Then, algorithms get skewed and machine learning exacerbates these problems, she added, providing many examples of bias against women in data, algorithms and machine learning – from banking to the job market, and beyond.
“Data tells a story. It’s more than just fields and values,” said Ms. Rogers. “My first step is to understand the data – how did it get to this place?”
We need to look at open, collaborative data sets and we need to reevaluate what’s in the original data, said Ms. Kraft-Buchman. “That’s important for policy makers,” she added.
Bringing ethics to the discussion
Panelists agreed on the importance of ethics in this discussion.
“If you’re going to release a new systems into the world, you need to think very hard about your social responsibility,” said Andy Coravos, CEO/Founder of Elektra Labs.
Kraft-Buchman mentioned that there should be a Hippocratic Oath for AI, similar to the one used in the medical profession to promise to work for the benefit of people.
“The challenge with Hypcratic Oaths is that people don’t remember them,” responded Ms. Coravos. “What’s better are checklists,” she said, giving examples of professions, such as pilots, where checklists must be followed in order to operate.
Ethics should be core and fundamental to technical classes for those learning how to create tomorrow’s AI solutions, offered Rogers.
“We need a really deep conversation about ethics and about what world we actually want to create,” said Tin.
What can be done on the policy side?
The public sector should consider setting aside funding for women-owned tech businesses with women-run teams of developers, said Kraft-Buchman.
“It’s not about creating a bunch of female Zuckerbergs,” she said, adding that women need to be working throughout the ranks of tech companies building the latest AI solutions. “It’s not only about making more unicorns or owning the company. We need to go broad and wide.”
“Policy makers need to have courage – and be encouraged – to ask questions about how the technology is made,” she said.
She also called for “a few less pizza-delivery apps” – and a few more solutions that using AI for social good.
There are so many big problems, but we are “somehow lacking the imagination to use tech to solve these problems,” she said. “Let’s use the tech to correct these.”
In the end, it’s not a technical problem, it’s a human problem, agreed panelists.
“What I’d like to see is a broader vision for who makes AI,” said Rogers. “You have to include people every step of the way. We have to put the human in the center of this entire process. We don’t have an algorithm crisis we have a crisis of caring. We have to care more about the people we’re creating solutions for.”