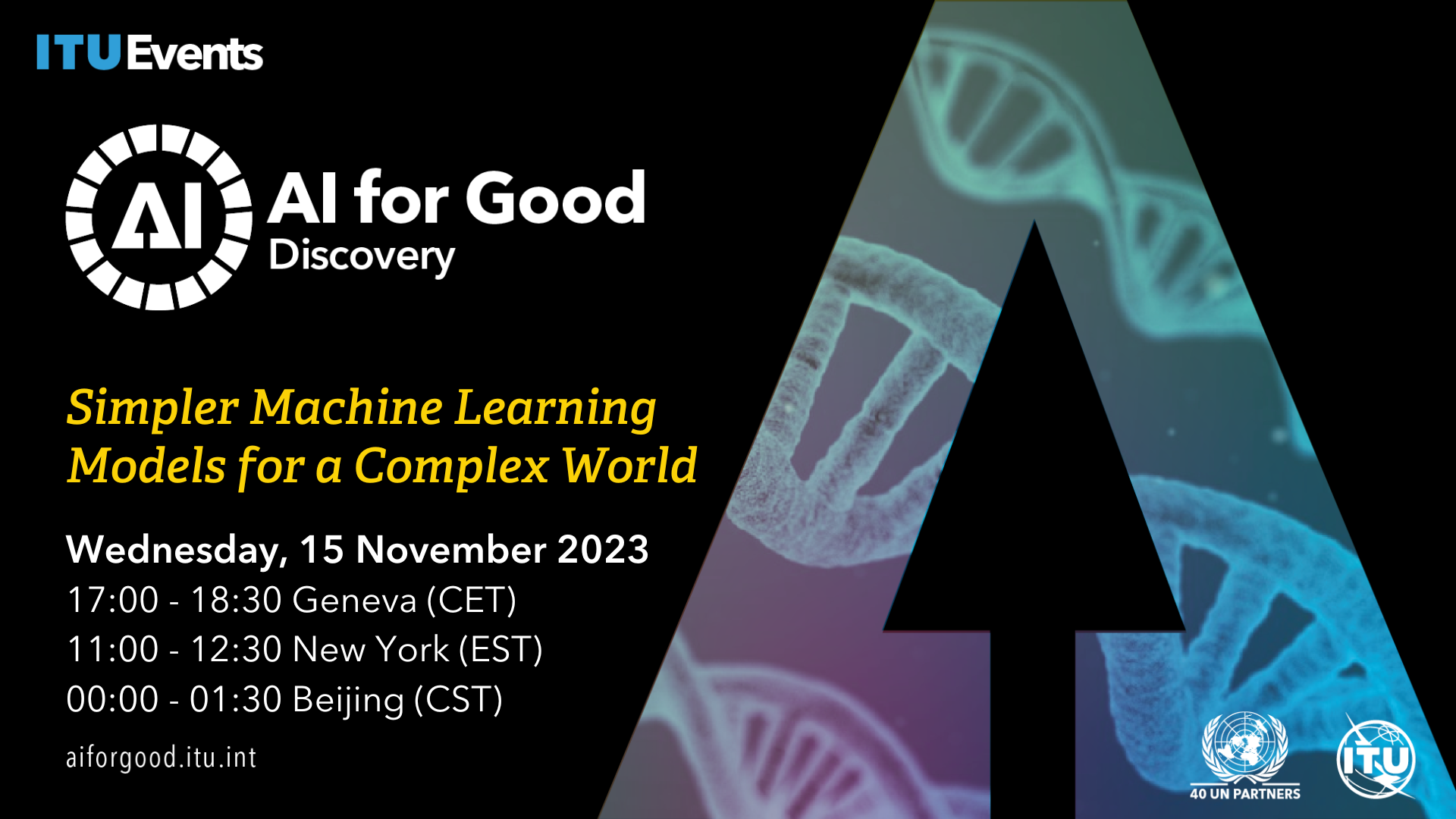
* Register (or log in) to the AI4G Neural Network to add this session to your agenda or watch the replay
While the trend in machine learning has tended towards building more complicated (black box) models, such models have not shown any performance advantages for many real-world datasets, and they are more difficult to troubleshoot and use. For these datasets, simpler models (sometimes small enough to fit on an index card) can be just as accurate. However, the design of interpretable models for practical applications is quite challenging for at least two reasons: 1) Many people do not believe that simple models could possibly be as accurate as complex black box models. Thus, even persuading someone to try interpretable machine learning can be a challenge. 2) Transparent models have transparent flaws. In other words, when a simple and accurate model is found, it may not align with domain expertise and may need to be altered, leading to an “interaction bottleneck” where domain experts must interact with machine learning algorithms.
In this talk, Prof. Rudin will present a new paradigm for machine learning that gives us insight into the existence of simpler models for a large class of real-world problems and solves the interaction bottleneck. In this paradigm, machine learning algorithms are not focused on finding a single optimal model, but instead capture the full collection of good (i.e., low-loss) models, which we call “the Rashomon set.” Finding Rashomon sets is extremely computationally difficult, but the benefits are massive. Prof. Rudin will present the first algorithm for finding Rashomon sets for a nontrivial function class (sparse decision trees) called TreeFARMS. TreeFARMS, along with its user interface TimberTrek, mitigate the interaction bottleneck for users. TreeFARMS also allows users to incorporate constraints (such as fairness constraints) easily.
Prof. Rudin will also present a “path,” that is, a mathematical explanation, for the existence of simpler yet accurate models and the circumstances under which they arise. In particular, problems where the outcome is uncertain tend to admit large Rashomon sets and simpler models. Hence, the Rashomon set can shed light on the existence of simpler models for many real-world high-stakes decisions. This conclusion has significant policy implications, as it undermines the main reason for using black box models for decisions that deeply affect people’s lives.
Prof. Rudin will conclude the talk by providing an overview of applications of interpretable machine learning within my lab, including applications to neurology, materials science, mammography, visualization of genetic data, the study of how cannabis affects the immune system of HIV patients, heart monitoring with wearable devices, and music generation.
This is joint work with Prof. Rudin’s colleagues Margo Seltzer and Ron Parr, as well as their exceptional students Chudi Zhong, Lesia Semenova, Jiachang Liu, Rui Xin, Zhi Chen, and Harry Chen. It builds upon the work of many past students and collaborators over the last decade.
Below are the papers Prof. Rudin will discuss in the talk:
Rui Xin, Chudi Zhong, Zhi Chen, Takuya Takagi, Margo Seltzer, Cynthia Rudin
Exploring the Whole Rashomon Set of Sparse Decision Trees, NeurIPS (oral), 2022.
https://arxiv.org/abs/2209.08040
Zijie J. Wang, Chudi Zhong, Rui Xin, Takuya Takagi, Zhi Chen, Duen Horng Chau, Cynthia Rudin, Margo Seltzer
TimberTrek: Exploring and Curating Sparse Decision Trees with Interactive Visualization, IEEE VIS, 2022.
https://poloclub.github.io/timbertrek/
Lesia Semenova, Cynthia Rudin, and Ron Parr
On the Existence of Simpler Machine Learning Models. ACM Conference on Fairness, Accountability, and Transparency (ACM FAccT), 2022.
https://arxiv.org/abs/1908.01755
Lesia Semenova, Harry Chen, Ronald Parr, Cynthia Rudin
A Path to Simpler Models Starts With Noise, NeurIPS, 2023.
https://arxiv.org/abs/2310.19726
This live event includes a 30-minute networking event hosted on the AI for Good Neural Network. This is your opportunity to ask questions, interact with the panelists and participants and build connections with the AI for Good community.