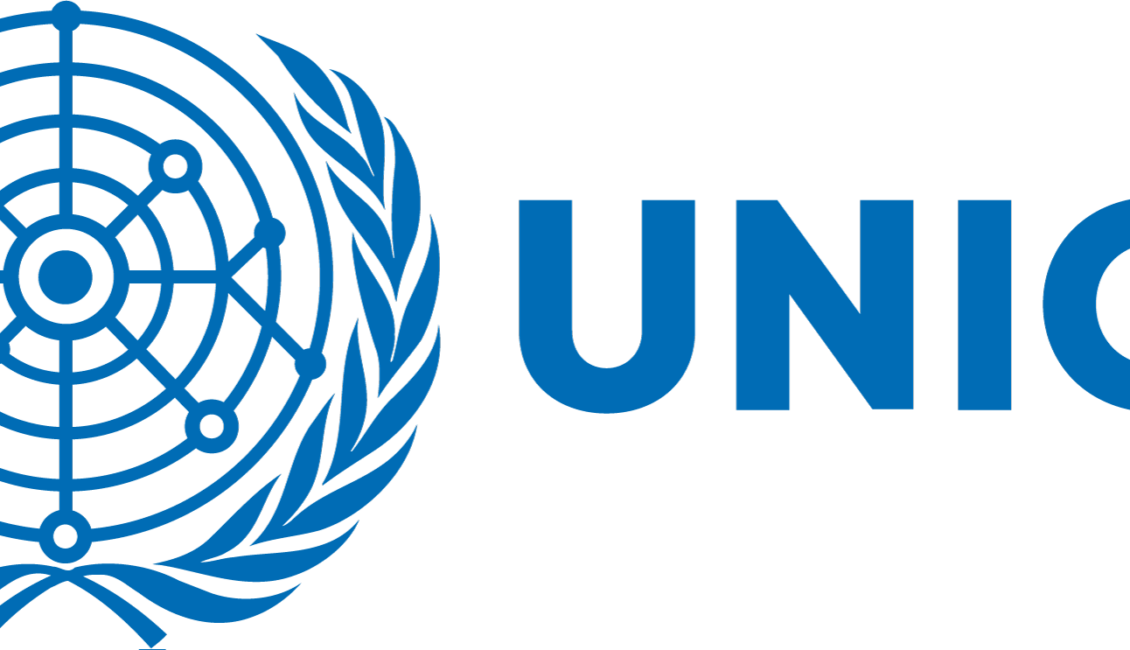
The United Nations International Computing Centre (UNICC) has over 50 years of experience as the largest strategic partner for digital solutions and cybersecurity within the United Nations system. We design and deploy transformational digital tools and programmes to support over 90 partners in fulfilling their mandates.
Description of Activities on AI
Project 1: An AI Approach to Flag Sexist Text Content on Social Media Channels in Latin American Countries
Rapidly growing access and use of information and communication technologies (ICT), accelerated by the COVID-19 pandemic, has had multiple impacts on gender equality and women’s rights, including the further exacerbation of existing forms of sexism, abuse and violence against women (VAW). At the same time social media platforms, especially X (Previously Twitter), have become powerful conduits for communication in Latin American countries. The rise of online sexism and the perpetuation of harmful narratives necessitate proactive measures.
To address this concern, UNICC and UN Women developed an artificial intelligence (AI) model designed to automatically detect and flag sexist text content on X (Previously Twitter) across Spanish-speaking countries in Latin America.
The AI model employs advanced supervised algorithms, carefully chosen and trained on diverse labeled datasets, to identify sexist and abusive language that may contribute to perpetuating harmful stereotypes. Recognizing the linguistic and cultural diversity across Latin American countries, the model incorporates a multilingual approach, considering regional nuances to ensure accurate detection.
Despite linguistic and cultural challenges, the model demonstrates promising results in detecting and categorizing sexist content. Continuous refinement and ethical considerations remain integral aspects of the approach we used, emphasizing the importance of flagging the content with freedom of expression.
Ultimately, the AI model serves as a valuable tool for addressing online sexism in Latin American countries. Its insights and trends gleaned from the analysis will play a pivotal role in informing the development of a systematic approach to prevention and response to sexism and technology-facilitated Violence Against Women (TF VAW). This includes evidence-based prevention interventions aimed at transforming harmful social norms, enhancing understanding of the issue in the region, and fostering the creation of safe online spaces for women and girls. Moreover, the model will contribute to the development of effective enforcement mechanisms and consistent standards for content moderation, specifically tailored for UN Women initiatives.
Lessons Learned
Effectiveness of OpenAI/GPT in Data Translation: The application of OpenAI/GPT in data translation has proven highly effective, maintaining exceptional standards of quality and performance.
Successes and Limitations of Keyword Approach: Leveraging keywords, we successfully identified sexist tweets with a detection rate of 10-12%. However, this approach fell short in fully distinguishing between different types of sexism.
Insights into the Relationship Between Sexism, Emotions, and Hate Speech: Our research has provided valuable insights into the complex relationship between various forms of sexism, emotions, and hate speech, enriching our understanding of these interconnected phenomena.
Competitiveness of Our Model: Despite the limitations, our model’s performance is comparable to other Spanish language models, highlighting its competitiveness within the field.
Areas for Improvement and Expansion: Moving forward, we have identified areas for improvement and expansion. Initiatives such as implementing a dashboard and conducting hyperparameter search are underway to enhance efficacy and efficiency. Additionally, we aim to broaden the project’s scope by adapting the methodology to encompass Spanish-speaking and other LATAM countries, facilitating code reutilization and scalability.
Potential Applications: Our research findings pave the way for several potential applications. These include the development of a real-time app to alert users about sexist content, as well as the creation of an API for seamless integration of our models into existing systems, benefiting stakeholders such as UN agencies and LATAM countries.
Overall Impact and Contribution: Through these endeavors, we aim not only to advance the field of AI-driven data translation but also to contribute to societal well-being by combating harmful online behavior and fostering a safer online environment.
Project 2: An AI Approach to Incident Management
The United Nations International Computing Centre (UNICC) is the leading digital service provider within the UN system. UNICC’s AI Approach to Incident Management integrates Information Technology Infrastructure Library (ITIL) processes, providing a structured framework for efficient incident management and problem resolution. This innovative approach aims to establish a robust decision support mechanism, refining the monitors responsible for initiating incidents. By enhancing the accuracy and effectiveness of incident detection, our approach contributes to more streamlined infrastructure management. Through the application of AI technologies, we strive to optimize the monitoring process, ultimately minimizing downtime and improving overall operational efficiency.
Lessons Learned
Text Cleaning and Data Anonymity: Our journey began with the critical step of text cleaning, focusing on enhancing data anonymity and confidentiality. Named Entity Recognition (NER) techniques were instrumental in removing proper names, while word embeddings tailored to the IT domain effectively captured domain-specific semantics. Additionally, refinement of stopwords and exploration of automatic techniques like NER for identifying organization names were vital in ensuring data quality.
Prioritization Strategies: We underwent an iterative process of experimenting with prioritization strategies. While some approaches initially seemed promising, we encountered roadblocks that hindered the classification process. Eventually, we settled on a method that involved reducing the number of classes from five to three and modeling the problem as binary classification. This decision allowed us to distinguish critical from non-critical incidents effectively.
Custom Vocabulary Development: A key insight gained was the importance of curating a custom vocabulary comprising words specific to high-priority incidents. This focused approach facilitated the development of a more accurate and impactful model.
Optimizing Data Analysis Workflows: Through the implementation of comprehensive processing and prioritization methods, our aim was to optimize data analysis workflows. By deriving actionable insights and driving informed decision-making, we sought to enhance organizational efficiency in incident management.
Culmination of Industry Standards and Iterative Experimentation: Our approach represents a culmination of industry-standard practices and iterative experimentation. By leveraging established methodologies and continuously refining our techniques through experimentation, we have developed a robust and effective methodology for data analysis in incident management.
By sharing these lessons learned and insights gained, we hope to assist others in navigating similar challenges and achieving success in their endeavors.
Project 3: Detection of Misinformation in Social Media
The Detection of Misinformation in Social Media project was a collaborative effort conducted between UNICC and NYU Capstone participants from 2021 to 2022. The objective of this joint endeavor was to leverage AI technology to develop a precise and efficient classification system for identifying “fake news” (i.e. misinformation and disinformation) encompassing misinformation and disinformation. The overarching goal was to empower users to assess the reliability of information encountered on social media platforms.
The term fake news encompasses both misinformation and disinformation, disseminated primarily through dedicated fake-news outlets, which purposefully fabricate and spread false information (Janice & The Verified Initiative of the United Nations, 2021). Misinformation constitutes unintentional errors, encompassing inaccuracies in statistical data, images, or comments that are mistakenly perceived as accurate. On the other hand, disinformation involves the deliberate creation or manipulation of audio or visual content, along with the propagation of intentionally crafted conspiracy theories or rumors, all aimed at gaining some form of advantage. Furthermore, disinformation can serve to suppress alternative viewpoints or divert attention elsewhere.
To address this pervasive issue, UNICC collaborated with NYU Stern (students &faculty) to develop a comprehensive data labeling approach aimed at categorizing information based on its veracity. Together, we established the following classification criteria:
“True”: Claims that have undergone rigorous fact-checking and are confirmed to be entirely accurate by credible sources.
“Misleading”: Claims containing varying degrees of falsehood, as determined by fact-checkers, including partial falsehoods, questionable assertions, or misleading information.
“False”: Claims that have been thoroughly debunked and proven to be entirely untrue by reliable fact-checking sources.
“Unproven”: Claims lacking sufficient evidence or scientific support to determine their veracity, categorized as unproven, unsupported, or unfounded.
This collaborative effort resulted in a structured approach to classifying content, enabling more accurate identification and assessment of information accuracy and reliability.
This AI based systematic classification was aimed at enabling users to discern the reliability of information they encounter, empowering them to make more informed decisions and combat the spread of misinformation and disinformation. By fostering transparency and accountability in the dissemination of information, this project’s approach can play a crucial role in safeguarding the integrity of public discourse and promoting a more discerning and critical approach to media consumption.
Lessons Learned
Enhancements in Dataset: Significant improvements were noted in our dataset, which now comprises a broader array of attributes for comprehensive analysis. With over 350,000 tweets collected for this project, each accompanied by relevant attributes, we have gained a rich repository of data to draw insights from. Despite fields like location and country remaining largely empty, their absence does not impact our analysis, given our focus on English-speaking tweets.
Impact of COVID-19 Pandemic on Twitter Activity: A noteworthy observation was the sharp increase in Twitter account subscriptions coinciding with the onset of the COVID-19 pandemic. This phenomenon suggests a mix of established institutions creating accounts during Twitter’s early years and opportunistic users capitalizing on the pandemic.
Effective Use of CRISP Methodology: The CRISP methodology for data mining proved to be effective in guiding our analysis efforts. This structured approach enabled us to efficiently navigate the complexities of the dataset, extracting meaningful patterns and insights.
Commitment to Robust Methodologies: As we continue to explore the data and its implications, our project remains committed to leveraging robust methodologies and innovative approaches to uncover actionable insights. We are continuously seeking new methodologies and techniques to enhance our analysis and drive informed decision-making.
Future Plans for Expansion: Moving forward, we are exploring the possibility of trying other AI methodologies and techniques to further enhance our analysis. Additionally, we are actively creating new annotated datasets related to social media to expand our research further and unlock additional insights in this domain. This commitment to continuous improvement and expansion reflects our dedication to delivering tangible benefits through our project.